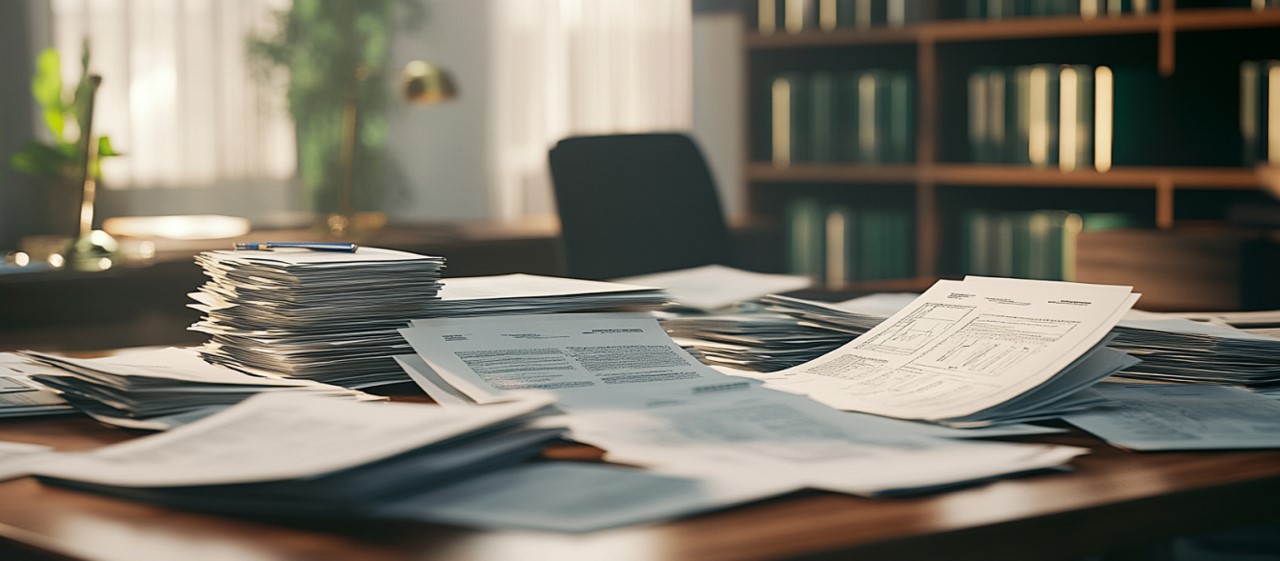
The Traditional Approach
Patent👉 A legal right granting exclusive control over an invention for a limited time. searching has long been a cornerstone of intellectual property👉 Creations of the mind protected by legal rights. management. Historically, this process was entirely manual, relying on the expertise of patent attorneys and researchers who meticulously combed through physical documents and archives. The traditional approach involved identifying relevant patent classifications and keywords related to an invention👉 A novel method, process or product that is original and useful., followed by a labor-intensive search through patent gazettes, technical journals, and other printed materials.
As patent offices expanded globally, so did the volume of patent documents. For instance, the United States Patent and Trademark👉 A distinctive sign identifying goods or services from a specific source. Office (USPTO) has issued over 11 million patents since its inception in 1790. This dramatic increase made manual searches increasingly challenging and prone to oversight.
While human-centric searches had their strengths—such as nuanced understanding of patent language and the ability to interpret complex technical concepts—they were often limited by time constraints and geographical scope. Conducting a comprehensive global patent search👉 A systematic review of patents literature. was prohibitively expensive and required access to multiple national databases, often necessitating foreign language skills.
The AI Revolution
The rise of digital technology in the late 20th century marked a pivotal shift in patent searching. Online databases emerged, significantly improving access to patent information. However, the true revolution came with the introduction of artificial intelligence (AI) tools in the early 2000s. These tools promised to transform patent searches by leveraging natural language processing (NLP) and machine learning algorithms to analyze vast amounts of data quickly.
AI-powered semantic search engines could understand context and meaning behind queries, allowing for more accurate results than traditional keyword-based searches. This capability enabled researchers to find relevant prior art that might have been overlooked using conventional methods.
As AI technology advanced, it began to automate tasks like document classification and clustering, making it easier to navigate large datasets. The excitement surrounding these tools led many in the intellectual property community to view them as solutions to the challenges posed by an increasingly complex patent landscape.
The Reality Check
Despite the initial enthusiasm for AI-powered patent search tools, their limitations soon became apparent. While these systems excelled at processing data rapidly, they often lacked the nuanced understanding that human experts brought to the table.
One major challenge was the quality of search results. Early AI algorithms sometimes produced overwhelming numbers of false positives, particularly in complex technical fields where subtle differences in terminology could significantly impact relevance. Additionally, AI struggled to grasp the inventive concept behind patents; it could analyze text but often failed to understand the underlying problem an invention aimed to solve.
Legal complexities also posed challenges for AI systems. Patent law is intricate and varies across jurisdictions. AI tools often lacked the ability to interpret legal nuances or apply recent case law effectively, which could lead to critical oversights.
Moreover, AI systems faced difficulties in handling non-patent literature—essential for comprehensive prior art searches. Scientific papers and technical disclosures often contain valuable information but are less structured than patents, complicating their analysis by AI.
As these limitations became evident, professionals began to shift their perspective. Rather than viewing AI as a replacement for human expertise, many recognized the potential for a complementary relationship between human intelligence and artificial intelligence.
Recognition of the Continued Need for Human Expertise
The realization of AI’s limitations led to a renewed appreciation for human contributions in patent searching. Human experts possess several irreplaceable qualities:
- Contextual Understanding:
Humans can grasp broader contexts around inventions, allowing for more targeted searches. - Creative Thinking:
Experienced researchers can make intuitive connections that may elude AI systems. - Legal Expertise:
Patent attorneys understand complex legal requirements and can interpret results accordingly. - Quality Control:
Human experts critically evaluate search results, filtering out irrelevant findings. - Strategic Decision-Making:
Humans can adapt search strategies based on initial results, refining queries as needed.
This recognition led to hybrid approaches that combine AI’s processing power with human analytical skills. In this model, AI tools conduct initial broad searches and identify potential matches while human experts review and refine these results based on their judgment and expertise.
Achieving Optimal Results Through Collaboration
The collaborative approach has proven more effective than either AI or human-only searches alone. It allows for efficient processing of vast patent databases while maintaining high accuracy standards essential for critical patent decisions.
Best practices for this collaboration include establishing clear roles within teams and developing training programs that equip patent professionals with skills to leverage AI tools effectively.
Case studies have shown that organizations employing hybrid models experience significant improvements in both efficiency and accuracy during their patent searches. For example, companies that integrated AI into their workflows reported faster turnaround times for prior art searches while maintaining high-quality outcomes.
As we look toward the future, ongoing advancements in AI technologies promise even greater enhancements in patent searching capabilities. Innovations in natural language processing and machine learning will continue to improve how we analyze patent data.
However, lessons learned from early experiences with AI have instilled a balanced perspective within the industry. The future of patent searching lies not in replacing human expertise but in thoughtfully integrating these two powerful resources.
By leveraging both human intelligence and artificial intelligence effectively, we can create more efficient, accurate, and comprehensive patent search processes that drive innovation👉 Practical application of new ideas to create value. while protecting intellectual property in an increasingly complex technological landscape.